High-performance Filters for Modern Hardware (GPUs) and Workloads (Adaptive).
Apr 27, 2022
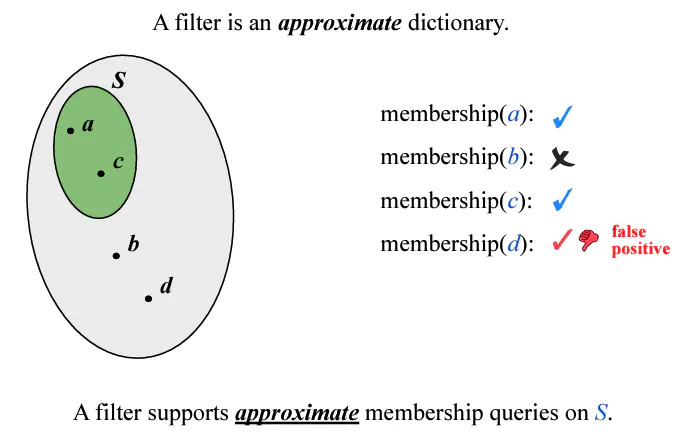
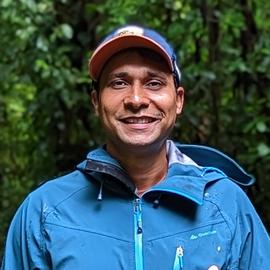
Prashant Pandey
Assistant Professor
My research interests lie at the intersection of Systems and Algorithms. I design and build theoretically well-founded data structures for big data problems in computational biology, streaming, and file systems.
Publications
Richard Wen,
Hunter McCoy,
David Tench,
Guido Tagliavini,
Michael Bender,
Alex Conway,
Martin Farach-Colton,
Rob Johnson,
Prashant Pandey
Prashant Pandey,
Martin Farach-Colton,
Niv Dayan,
Huanchen Zhang
Hunter McCoy,
Steven Hofmeyr,
Katherine Yelick,
Prashant Pandey
Madhav Narayan Bhat,
Paul Cesaretti,
Mayank Goswami,
Prashant Pandey
Prashant Pandey,
Alex Conway,
Joe Durie,
Michael Bender,
Martin Farach-Colton,
Rob Johnson
Mayank Goswami,
Dzejla Medjedovic,
Emina Mekic,
Prashant Pandey
Prashant Pandey,
Michael A. Bender,
Rob Johnson,
and Rob Patro
Prashant Pandey,
Michael A. Bender,
and Rob Johnson